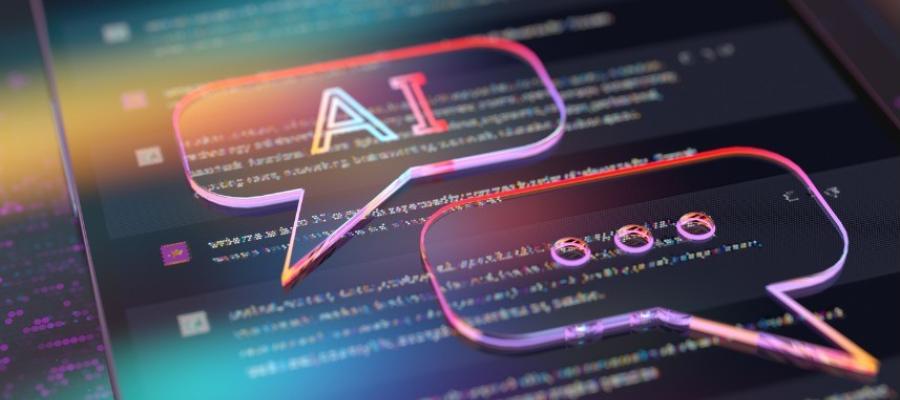
PODCAST
An Evolving Landscape: Generative AI and Large Language Models in the Financial Industry
Generative Artificial Intelligence (AI) and large language models (LLM) are taking the world by storm, presenting numerous opportunities to create business efficiencies. While the new technologies offer many potential benefits to firms, regulators and investors, they also introduce unique risks.
On this episode, we hear from Brad Ahrens, senior vice president of Advanced Analytics, Andrew McElduff, vice president with Member Supervision's Risk Monitoring team and Haime Workie, vice president and head of FINRA's Office of Financial Innovation, who are closely looking at these technologies and following developments in this space to learn how FINRA is looking at and thinking about generative AI when it comes to its own business and what it's looking at and seeing when it comes to firm use of these tools.
Resources mentioned in this episode:
Artificial Intelligence (AI) in the Securities Industry
Artificial Intelligence (AI) and Investment Fraud
Reg Notice 21-19: Obligations Related to Outsourcing to Third-Party Vendors
2024 FINRA Annual Regulatory Oversight Report
2023 Executive Order on Artificial Intelligence for Congress
Listen and subscribe to our podcast on Apple Podcasts, Google Podcasts, Spotify or wherever you listen to your podcasts. Below is a transcript of the episode. Transcripts are generated using a combination of speech recognition software and human editors and may contain errors. Please check the corresponding audio before quoting in print.
FULL TRANSCRIPT
00:00 - 00:32
Kaitlyn Kiernan: Generative AI and large language models are taking the world by storm, presenting numerous opportunities to create business efficiencies. While the new technologies offer many potential benefits to firms, regulators and investors, they also introduce unique risks. On this episode, we hear from three experts at FINRA who are closely looking at these technologies and following developments in this space to learn how FINRA is looking at and thinking about generative AI when it comes to its own business and what it's looking at and seeing when it comes to firm use of these tools.
00:32 – 00:41
Intro Music
00:41 - 01:15
Kaitlyn Kiernan: Welcome to FINRA Unscripted. I'm your host Kaitlyn Kiernan. I'm excited to have three guests with us today to talk about a topic that feels like it's going to be the topic of 2024, and that is generative AI and large language models. Joining me today to dig into this new and evolving space are FINRA Unscripted first-timer, Brad Ahrens, senior vice president of Advanced Analytics, and repeat guests with us, Andrew McElduff, a vice president with Member Supervision's Risk Monitoring team and Haime Workie, vice president and head of FINRA's Office of Financial Innovation. Brad, Andrew and Haime, thanks for joining me today.
01:16 - 01:16
Haime Workie: Glad to be here.
01:17 - 01:17
Andrew McElduff: Thank you.
01:17 - 01:29
Kaitlyn Kiernan: Just to kick us off, can you start by introducing yourselves? Brad, since you are newer to FINRA and FINRA Unscripted, can we start with you? What's your background and what did you do prior to joining FINRA?
01:29 - 02:06
Brad Ahrens: Yes, thanks for having me. I started in the brokerage industry about 30 years ago, and for about 24 years worked in compliance at a very large retail firm on the street, mainly in surveillance, analytics, sales practice, financial crimes, enterprise risk management with a touch of regulatory examinations and inquiries. During that time, I was really focused on bridging the gaps between compliance, regulation, business and technology. We also started doing a fair amount of data mining and analytics within compliance, whether it was for a regulatory exam or inquiry or to better inform us about what our business was engaged in.
02:07 - 02:16
Kaitlyn Kiernan: It sounds like you have some familiarity with some of the challenges facing firms, but how else do you think your previous experience prepared you for your current role?
02:17 - 02:57
Brad Ahrens: Analytics is all about generating outcomes for the end customers, whether you're at a firm or at a regulator. So, really, we've been focused on, here at FINRA and at my prior firm, making sure we've had the right tools, the right data scientists, the right data engineers and, most importantly, business side subject matter experts that could connect all the dots between what the business is doing, the regulatory environment and everything else that was happening within the industry. So, being in the industry for so long exposed me to all sorts of technology, data, business practices, market busts and booms and that's been a great help in coming to FINRA and working with all the partners here.
02:58 - 03:09
Kaitlyn Kiernan: Andrew, we had you on the show about a year ago when we were introducing the Risk Monitoring team. Can you remind us what you do with Risk Monitoring and how that all ties in with the topic at hand today?
03:09 - 03:55
Andrew McElduff: Yeah, thanks, Kaitlyn, and pleasure to be back with the podcast. So, still the same role with Risk Monitoring. I'm the Vice President and head of the Retail Risk Monitoring team here at FINRA, focusing on about 1,200 firms across the retail space. And I partner with our other VPs in Capital Markets, Diversified, Trading Execution and Carrying & Clearing. About a year ago, we were talking about the changes and the evolution of Risk Monitoring and our transformation and our structural changes. All of those changes have led to this world that we're in now, where we can better focus on and know more quickly what's happening at our member firms. And no better topic than AI and especially generative AI and LLMs. Risk Monitoring is trying to play a critical role at FINRA in outreach to member firms, different techniques of outreach, and soliciting and gathering back information in this space.
03:56 - 04:05
Kaitlyn Kiernan: Thanks, Andrew. Haime, I haven't counted, but you've been on a number of episodes of FINRA Unscripted with us. But can you remind us what you and the Office of Financial Innovation do?
04:06 - 04:41
Haime Workie: I'm glad to have the opportunity to be back on the podcast. For those that may not have heard me on a prior podcast, I'm Haime Workie and I work within FINRA's recently formed Office of Regulatory Economics and Market Analysis where I head up the Office of Financial Innovation, or OFI for short. OFI is really designed to facilitate innovation in a way that's consistent with FINRA's broader mandate, which, as many of you know, is investor protection and market integrity. As part of these efforts, we've been focused on issues related to artificial intelligence for a number of years now, and those efforts have recently been enhanced as a result of the developments related to generative AI.
04:42 - 04:55
Kaitlyn Kiernan: Thanks, Haime. So, before we dig in, I wanted to start out by noting the date. We are recording this at the end of February for posting in early March. And this is important because this is an area that's quickly evolving.
04:55 - 05:12
Brad Ahrens: Yeah, there's actually a fair chance that this could be out of date in the next two weeks, but we'll provide a high enough overview to give the general direction of where things are headed. And we always can be surprised with what pops up in the marketplace and generative AI in terms of uses and new features and capabilities that will be rolled out.
05:13 - 05:36
Kaitlyn Kiernan: So, with that caveat aside, we'll dig in. When OpenAI launched ChatGPT in November 2022, it took the world by storm. But we're more than a year into this, and I think there's still confusion around what this technology is or isn't. So, Haime, can you help us by explaining some key terms, including what is artificial intelligence or AI?
05:37 - 06:22
Haime Workie: So, artificial intelligence is really an umbrella term. It encompasses a broad spectrum of different technologies and techniques, but at its core, AI is designed to have machines perform functions. They're designed to imitate intelligent human behavior. There's a variety of different AI techniques, including things like machine learning, where you can train a machine to recognize patterns and provide output based on prior data sets. There's also deep learning, which involves using neural networks to identify patterns, and other techniques like natural language processing, computer vision, and the one that's probably generated the most interest recently, generative AI. It's important to understand the specific technique that's being used in artificial intelligence if you want to understand the potential benefits and challenges that are being posed.
06:22 - 06:27
Kaitlyn Kiernan: And how has FINRA been involved with AI, generally, to date?
06:28 - 08:26
Haime Workie: A few years back, FINRA published an AI report that highlighted the implications of AI for the securities industry more broadly. The report contains several key takeaways, one of which was that broker-dealers are largely using AI-based tools in three areas: communications with customers, the investment process and operational functions. With respect to communications with the customers, this can include things like chatbots, which are probably the ones that are the most familiar to people, but also working with, kind of, virtual assistants, for example, things like Google Home or Amazon Alexa to be able to do things like find out what my balance is on my account.
In terms of the investment process, this includes things like trading as well as portfolio management. With respect to trading, you can have AI systems that are designed to gain information based off of alternative data sets or different types of data and feed that into the trading decision. They could also have the AI being used in the context of the trading itself in order to do things like help determine the platform for best execution.
Another key takeaway from the report was that AI presents several unique challenges. A couple of those include things like explainability, the ability to be able to have an understanding of how the machine came to a result. This is important, particularly in the context where AI is being deployed directly to customer facing products. Another important area in terms of unique challenges include things like data bias. So, this could be things like statistical bias where you have oversampling or under sampling, but also can include things like demographic-related biases where you may have decisions, for example, in the machine learning context that were made based off of old biases that may exist, for example, with things like redlining, where if you're not conscious of those biases in the initial set of decisions where you're using tools like machine learning, you can actually exacerbate that. And then finally, I would just note that in addition to some of these challenges, AI obviously provides a number of different potential benefits for investors and for the firms themselves.
08:28 - 08:45
Kaitlyn Kiernan: Thanks Haime. So, the topic that is more recently emerged is the generative AI, that's the 'G' in ChatGPT. Brad, what is generative AI and how does it differ from earlier forms of artificial intelligence that Haime mentioned?
08:46 - 10:08
Brad Ahrens: So, generative AI uses new foundational models to allow users to create and generate content such as text, images, audio and video. The new models have been trained on massive, massive data sets to learn patterns and relationships within that data. And those data sets are just huge in terms of size, scope and scale, and include most of the data that's found on the internet. Both the good and the bad of the internet has all been used to train the new models. So, once the models are trained, users can leverage the models by prompting the model with questions and commands that can be written in plain English almost conversationally, as opposed to the older, more traditional models.
The generative AI capabilities are expanding, as we noted, at a very rapid pace, so you could use the models to generate images, create content, such as a report or let's say, a business plan or even a school paper. You can use them to summarize documents, classify all sorts of data, help with your kid's math homework, assist in code generation, and the list just goes on and on from there. What we're seeing emerge also is using generative AI to act as an agent for you, where it can execute some pre-commanded instructions to help create efficiencies in ongoing repetitive processes.
10:09 - 10:17
Kaitlyn Kiernan: Yeah, that's really interesting. And so, as a related question, what is a large language model and how is that new and innovative?
10:17 - 12:36
Brad Ahrens: It's a bit in the name. So, it uses large and it's all about language. A large amount of data is used to train these models, and it's really focused on language or text. The large language models differ from a lot of the earlier machine learning models. So, a few things have happened in about the past six or seven years. First, around 2017 or so, there was a significant innovation in machine learning models that introduced something called a transformer. So, a transformer works when applied to words and text, for example, by allowing the logic within the models to learn the context of the words by tracking the relationship between the words or sentence in a paragraph. That means that a model will be far more accurate in predicting the next word in a sentence.
So, if I use an example sentence that says, "the chef baked a meringue pie using the following ingredients," an old model might have a very hard time figuring out an ingredient. It might even say the word "pasta." A newer, large language model that's using transformers within it will likely generate the list of accurate ingredients that are in a meringue pie. It could say lemon juice, flour, water, sugar, eggs, egg whites, et cetera and it will be a lot more accurate. Now how does it do that? Well, the second most important thing here is that the amount and scale of the data that's used to train the latest generative AI models is far greater than has ever been used in traditional machine learning models. A newer model, like GPT-4 is pre-trained on over a trillion different parameters.
Older models, even the very early model or the early versions of GPT were trained on, let's say, maybe a few million parameters. And now, as you can see in less than five years, the model companies are now using 100 times the amount of data to train the models. The last thing here is that the new generative AI models are generative. They generate outputs that again, text, sound, images, that closely resemble the same patterns in relationships found in the training data. That wasn't the case with old machine learning models at all. So, that's why new large language models are so innovative and can be used in various spaces across not only the brokerage industry, but any other industry that's out there.
12:37 - 12:53
Kaitlyn Kiernan: You mentioned some examples that I think we've all seen people having these tools write essays or helping with homework or coming up with the recipes. But Andrew, what is the industry looking at and thinking about when it comes to these technologies? Probably not writing recipes.
12:54 - 17:39
Andrew McElduff: Well, now we all know that Brad is a baker, and we should all be contacting him for his family recipe. I'll take a step back to where we started on our outreach to educate ourselves on what the industry has been doing. In mid last year, Risk Monitoring started doing some outreach calls to a handful of firms to understand what was happening, what was the firm's position, what was their thought process, were they diving right in and trying to figure out how could they use these advances in technology? By and large, the feedback that we had gotten through that outreach of probably about 50 to 60 firms was firms were all interested, but they were taking a very conservative and dialed approach to understand what was the impact with the focus being on okay, new technologies, but if we were to use these, what's the risk to our firm and our clients?
Primary areas that we've discussed with firms and firms have raised with us is customer information protection, supervision, books and records, cyber related requirements and protections that have to be in place. So, all of that said, we then took that feedback. We met with teams like Brad and Haime internally and decided, what's the best way to advance here? We then, in November of 2023, issued a questionnaire to our membership more broadly about vendors that they're using so that we, as FINRA, could be better positioned to understand if or when there is an event related to a third-party vendor. How can we speed up our response and our proactive outreach to member firms? We understand that you're using this vendor. We understand that this is an issue or a breach that's happening.
Within that questionnaire, we did include a single question, but a two-part question related to generative AI and LLMs. The questions focused on vendor supported artificial intelligence. We did limit that question to generative AI and large language models. The second part of the question was open source or internally developed and supported artificial intelligence tools. So, we tried to aim it at both vendor as well as internal and or open source, similar to your ChatGPTs, where you can get it on the open-source market. So, I should make sure that I give a shout out to the membership. As of last week, we were at a 99.7% response rate on that questionnaire. So, thank you to the industry, all the folks that have contributed back to that.
From that information, what we're starting to see is the biggest and most powerful implementation we're seeing so far is efficiency gains. How can firms, as they look at this, build out processes or tools to support their processes, question and answer retrieval for firms with procedure manuals that could be a couple hundred pages long or employee handbooks, how can you ask a question of a tool and get an immediate response back? Obviously trained on all internal related information, thereby limiting the skew or the hallucination risk there? More broadly, we're also seeing firms focus on a couple of different areas, again, to simplify the human processes. How can you have a tool review EDGAR filing, say a 10-K, extract the key pieces of information and push it back to the key parties within your organization. How can you have a tool listen to quarterly earnings calls, report that back, have a tool create presentations, create PowerPoints based on a set of data that you would like included, up to and including having an avatar speak on the topic.
And I will give a shout out for the FINRA Cybersecurity Conference. Within that conference, they played two different recordings of an individual reading a sentence. One was a generative model. The other one was the person actually reading into a microphone. And I'll say, sitting in the audience, I could not tell the difference until pretty close to the end of the recording, where the model really started to fail. So, again, similar here, where you don't have to have a human speaking, but how can you train a model to then create that voice over? We're also seeing and have heard from firms on surveillance mechanisms. How can you train these models and get a human down to a subset of information, versus reviewing more broad or massive information sets such as e-communications, trading information, and other supervisory related functions.
And then finally, the last piece, and I think Brad had touched on this a little bit earlier, is code generation, all these things that will then help to ease the burden on the respective firms and their technology teams, or their third-party vendors that are performing those functions for them. Obviously, I've oversimplified it. I've kept it to a couple high level topics, but the overall and most common theme that we've heard from our largest firms, down to some of our smallest firms that are wading into this area, is a very, very conservative and dialed approach. And I would say the largest piece of feedback we have heard from firms as they start to work on this is we are not using customer information, so they are making sure that they're well positioned on the front end before they even consider loading any customer information, ensuring all contracts are up to date and everything else is dialed in before they are comfortable touching anything related to customers and their confidential information.
17:40 - 18:00
Kaitlyn Kiernan: That's really interesting to hear. And a 99.7% response rate is very impressive for a survey. So, if you're one of the dozen or so firms that have not responded, uh, here's your reminder on that. But out of the firms that did respond, which is almost all of them, how many are looking at this technology?
18:02 - 18:47
Andrew McElduff: I would say right now it's still in the minority. Reminder the question was a two-part question of vendor driven as well as open source and internal. Without question, it's vendor driven that is the larger population in the space versus internally developed. I say vendor driven, but some are still using some open source but tag it as a vendor. We have approximately, I'd say just over 100 firms that have affirmatively responded in this space, obviously some with multiple responses for different parts of their business or how they'd be using different models. So, I would say we're looking at probably about 75 to 80 unique firm related use cases in this space right now with that number continuing to grow and, going back again to the preamble, we asked this question and two weeks from now, I think it could be another 100 firms that are saying, you know what, we're in this space.
18:48 - 18:58
Kaitlyn Kiernan: Maybe after they listened to this podcast, the numbers might change. But what do firms need to know when it comes to supervising a vendor's use of these technologies?
19:00 - 20:00
Andrew McElduff: I don't see it as a generative AI/LLM related question, but I think it's more broadly a vendor management question. For any vendor that you have a contract with or a relationship with, ensure that contract protects you and your member firm and the information that you're responsible for, but also your clients. What are the give up rights? What's the information? Who's going to have a touchpoint to that information? Where will that information be stored? If you're loading it into a vendor's system, what can they use it for? Do you know that it's restricted only for your firm and your firm's use? And if not, make sure that you ask those questions as well as your opt out clauses and everything else related to that. So, on the more broader general vendor relationship and management cycle, and then asking yourself as a firm, what are we paying for and what are we getting? And then the last piece I'll mention is the breach relationship. If there's a breach or a system failure, how quickly are you notified? And just putting a plug out there for the SEC's rule in this space, and the prompt reporting of breaches and or any fails in a relationship there.
20:01 - 21:58
Brad Ahrens: Back in 2021, FINRA published a Regulatory Notice 21-29 on the topic of supervisory obligations related to outsourcing to third parties. We're going to find that few, if any, firms are going to be developing their own generative AI foundational models from scratch. When you look at the cost that's involved and the time and effort that's been involved, from the largest vendors that have created models that are now available in the marketplace, even the open-source models that are out there, it's going to be very unlikely that anybody builds their own. As such, a lot of people are going to be using a vendor here. They're going to be putting a vendor model into their environment and then using it for various activities as we described previously.
So, a few things apply here. And a lot of this is found in the Notice as well. Rule 3110, we'd expect member firms to develop reasonably designed supervisory systems appropriate to their business model and scale that would address technology governance around the AI. When it comes to AI and generative AI, firms really need to understand the risk and limitations. What data is being used in the model, the model layer itself, and then what are they doing to monitor that model over time through model monitoring? On the cybersecurity side, Reg SP is out there. Firms need to ensure their records remain secure and confidential at all times. Could your data leak with an AI model? It could. You really have to take a hard look at that and understand and ensure where the data is really going within the model. And then one thing to always consider is business continuity. If you're using an AI model for a specific part of your business and it starts to fail or it starts to drift like models can do over time, what's your plan there? Especially if it's used for critical business functions.
21:59 - 22:20
Kaitlyn Kiernan: When we were talking before this call, you also mentioned that sometimes you might have vendor risks where you don't expect them. Like you gave an example of a firm where it was just a video software company. They were doing virtual calls and they started doing AI transcripts. How does that kind of risk have to play in here? And what do firms need to look out for?
22:20 - 23:17
Brad Ahrens: What we're going to see in the marketplace, and we're already seeing it already with vendors, they're adding generative AI into their tool sets. It gives them an edge in terms of the capabilities and functionality within their tool, perhaps. And for many vendors, they may not always tell you that it's there in the tool. And could a vendor require you to opt out, turn the switch off or on whether you want to use it? Yes, there are some vendors that have turned on the functionality as soon as you did the upgrade, and a lot of firms have had to scramble to turn it off. The transcription is a very relevant example, but there's a lot of tools maybe in your HR department, maybe in your other operational tools that are coming down the pipeline that will have generative AI built right in. And you really have to ask the hard questions of the vendor, what's in their pipeline, what are they going to be delivering, what's in the roadmap? Does anything in the tool include AI and generative AI?
23:18 - 23:45
Haime Workie: You can delegate a function, but you can't delegate the ultimate responsibility. So, firms, particularly as Brad was alluding to, were using it in critical functions, for example, in helping a registered rep make a decision about an investment, that you're making sure that you have an understanding of how the tools that you're using, how they're coming up with decisions, that you have a model governance around those tools to make sure that you feel comfortable to taking on that responsibility.
23:47 - 23:58
Kaitlyn Kiernan: Thanks, Haime. So, we've talked a lot about how firms in the industry are looking at this technology. But Brad, what is FINRA doing to explore this technology for its own uses?
23:59 - 25:05
Brad Ahrens: Yeah. So, around April 2023, we formed up what we call the Large Language Model Coalition to bring together and leverage expertise from across the organization to basically explore, research and disseminate information and progress around the opportunities that are out there. Potential use cases, risks, challenges, and limitations presented by generative AI and specifically large language models. Our coalition is comprised of about 50 people from nearly all areas of FINRA. We've got people from Regulatory Ops, technology, cyber, Office of Chief Legal Counsel, OFI, Government Affairs, audit, Investor Education, HR. I think maybe I've missed one, but it's very, very large because we do need a lot of experts to come together to determine how can we use these and then where are the risks? Because a lot of the things that we're seeing right now are new—typically haven't bumped into those in typical software or other earlier, traditional machine learning models that we've used out there.
25:06 - 25:11
Kaitlyn Kiernan: And so, how would you describe FINRA's overall approach to exploring these technologies?
25:12 - 26:39
Brad Ahrens: Within the coalition, we formed up three primary working groups. The first one is the Internal and External Opportunities Working Group. We're really looking there for use cases within FINRA. And then also, as Andrew discussed earlier, how are members using large language models? We need to figure out not just where we could use them to improve efficiency and effectiveness, but also what are the key risks and challenges of those implementation and make sure that we're putting adequate policies and procedures and controls around there. The second group that we've got is the Technology Working Group, and they're largely working on the tech stack. What's going to be necessary to support the implementation and the experimentation using various models?
They're also heavily involved in assessing the cyber security risk, also grinding through contract language. And they've also started to build out some initial educational and training sessions for FINRA staff to take. And then last but certainly not least, is the Policy Group and our Contracts Group. They're drafting our internal usage policies both for end users and developers, our data scientists that are going to be implementing potential models here at FINRA. They're also working through all of our vendor contracts, both for the LLM providers and, as we just spoke on, other vendors who may now be including generative AI within their tool sets and embedding that functionality within.
26:40 - 26:51
Kaitlyn Kiernan: That's good to know. We have talked a lot about risks generally in this space, but are there any other risks around these new technologies that we missed?
26:52 - 28:19
Haime Workie: We covered a large number of the risks. I think there are some that we have discussed that may be worth highlighting a little bit more. I know we talked about explainability. We talked about data bias, we talked about data privacy. But in terms of data privacy, I think it's really important to understand the privacy of the data that you're potentially entering into the generative of AI models, but also the data sets that are being used to deploy outcomes or information that's being trained on the system. How that data information is being used when you're entering information into the system is really important, particularly if it contains customer information or it contains other types of proprietary information that you may want to not leak out, as well as the data sources that you're using for outputs, that you have a good understanding of that, so that the kind of responses you get back are the ones that you want.
And then cybersecurity risks—although we included this before, I think it's worth discussing a little bit more. There's been a lot of attempts to develop various generative AI based methods for cloning voices, as was discussed, cloning images, cloning texts. So, all these new ways of being able to have hacks or other types of cybersecurity risks that existed before, but potentially allows individuals or nefarious actors to exploit them in a more efficient manner, I guess, for lack of a better term, are things that people need to take into account when they're deploying things like systems to allow you to gain access to your account.
28:20 - 28:57
Andrew McElduff: Firms at this point probably need to reconsider or reevaluate as they do on a periodic basis. Something like a fund movement request in the past may have required written authorization from the client and a call back to the client. Can that be impacted now in this space, if a bad actor has access to both the written communication and a verbal response? How or what methods will you take to ensure that you're getting in touch with the client, and the true client themselves, before making that transaction happen, or that wire movement happen and funds are moved. The time of multifactor being as simple as a written response and a call back may now be impacted.
28:58 - 30:36
Brad Ahrens: A few other risks and challenges here. There can be toxicity within these models. They do try to put some guardrails into the models regarding toxicity of the responses that are going to come off of them, but bias would also include toxicity. So, could it reproduce offensive language or unfounded perspectives that are in the bad web content out there? That's quite possible. Another couple of things. Attribution models can always, in this space, tell you where the source for that specific information is. Now there are some improvements in capabilities retrieval. Augmented generation in a generative AI model is now available where you can get an answer and it can cite to the specific source, if you will, that is now available.
And then the last two things are hallucinations. Models, not all of them are very good at saying 'I don't know.' They do always try to give an answer to the person who's entering the prompt, and that just produces incorrect responses, where perhaps there's not enough training data out there so the models will start to hallucinate. And then the last thing finally is jailbreaking. So, models that are exposed to end users can be subject to adversarial use and adversarial prompting that tries to skirt and get around the implemented guardrails. And a lot of these challenges that Andrew has covered, Haime's covered and I've covered are not typically found all the time in either traditional model. Some are, some aren't, and a lot of these risks aren't in your usual software development.
30:36 - 30:55
Kaitlyn Kiernan: That's interesting. Thanks, Brad. And we have talked about a couple risks that do touch on individual investors. But what other risks do these technologies pose to the clients of the firms and the individual investors out there? And how can firms and regulators like FINRA work to address those risks?
30:56 - 32:34
Haime Workie: Well, Kaitlyn, I think that's a really important question because as I mentioned earlier, an important part of our mandate is investor protection. And FINRA, together with the Securities and Exchange Commission and the North American Securities Administration Association, or NASAA for short, recently issued an investor alert on artificial intelligence and investment fraud. The alert noted a number of different items. I'll just highlight a few of them, one of which is that investors should watch out for unregistered or unlicensed investment platforms claiming to use AI. We've actually seen a number of these proliferate since the release of ChatGPT by OpenAI, where you have these investment tools, some claim to provide information, some claiming to potentially do more, potentially give you advice, that are unregistered. And so, folks should really be wary of tools that are unregistered. And make sure you understand what the information is that you're getting before you make any type of investment decisions based off of it.
The other area the alert highlighted is that there's a number of different AI enabled technology investment scams, including the use of deepfakes or voice cloning, in order to give misleading or false information to entice people to make investment decisions frequently set up in the context of pump and dump or other types of schemes. And finally, I would just note that it's important not to rely solely on just generative AI based information in order to make investment decisions. It can be a factor, it can be actually an important input, but you should also be looking at other types of information and also your own investment financial needs before making those types of decisions.
32:35 - 32:59
Kaitlyn Kiernan: Thanks, Haime, and we'll link to that investor piece in the show notes as well. So, Andrew, you have mentioned you've had the survey out to firms. You've done a lot of proactive calls out to firms about their use of these technologies. As firms start getting involved in the space, what kind of information does FINRA expect to be receiving from firms as they explore AI?
33:00 - 34:36
Andrew McElduff: We're still in our maturation phase on our side too. So, as I mentioned, having just recently completed the vendor questionnaire where this was included, I think the what's next would start with Risk Monitoring. So, firms can and should anticipate follow up outreach from their Risk Monitoring Analyst, their Risk Monitoring Director, as well as folks from Brad's team. We want to have the experts facilitate the calls and have in-depth conversations with our membership. Those calls, and as you prepare for them, what to prepare for. What is FINRA going to ask about? I would start with the 2024 FINRA Annual Regulatory Oversight Report. The cybersecurity and the technology management section speaks to a lot of what we're going to be asking about. And Brad and Haime have touched on it here.
What's the technology that you're looking to use? Is it internal or is it via vendor? What contracts have you put into place? What considerations have you taken as part of that contractual process? Where are you in the process? What governance have you set up? What's your QA testing? What data are you using? How large is your corpus of data as well as ultimately the key question, what are you looking to get out of it? You started with a thesis and you want to try and see if that's going to work. Obviously, firms are spending a lot of time, money and resources on this. Why did you start down that journey and how will this improve the efficiency or the effectiveness of your member firm? And ultimately from, as Haime touched on, investor protection, how does this potentially impact that? So, I think those are the key questions to start thinking about preparing your responses on. We have, as Brad mentioned, a large number of data science teams and folks on our side that we can ultimately get into that granular conversation, but that's not where we want to start.
34:38 - 34:44
Kaitlyn Kiernan: Final question, Brad. Are there any other resources out there that firms should be aware of on this topic?
34:45 - 36:07
Brad Ahrens: Yeah. There's three primary areas that you can take a look at. First off, there was an executive order released back in late October that's really focused around the safe and secure and trustworthy development of end use of AI. It's worth a read because it's really focused on harnessing the AI for good use, non-adversarial use, and making sure that you're taking steps to mitigate the risks that AI and especially generative AI start to produce and that are out there. There's also NIST, which is the National Institute of Standards and Technology. It's got some really good guidance that they've now posted. They've got technical AI standards, an AI risk management framework that really focuses on how do you ensure that your AI is valid and reliable? How do you ensure it's safe? How do you ensure it's secure and resilient along with transparent and explainable as well as fair? And then NIST also has a playbook out that you can follow along with and really take a look at it when it comes to how you're managing your models in general, to make sure that you're applying the right sort of governance, that you're measuring things appropriately, and that overall, you're managing your AI in a way that really focuses on safety and security and trustworthiness.
36:08 - 36:45
Kaitlyn Kiernan: Well, that's it for today's episode of FINRA Unscripted. Thank you, Brad, Haime and Andrew, for joining me for what's our first but likely not our last episode about generative AI and large language models. Listeners, if you don't already, be sure to subscribe to FINRA Unscripted wherever you listen to podcasts to stay up to date on all our latest episodes, and if you have any ideas for future episodes or feedback on today's episode, you can email us at [email protected]. Today's episode was produced by me, Kaitlyn Kiernan, coordinated by Hannah Krobock and edited and engineered by John Williams. Until next time.
36:45 – 36:50
Intro Music
36:50 - 37:18
Disclaimer: Please note FINRA podcasts are the sole property of FINRA, and the information provided is for informational and educational purposes only. The content of the podcast does not constitute any FINRA Rule or amendment or interpretation to such rules. Compliance with any recommended conduct presented does not mean that a firm or person has complied with the full extent of their obligations under FINRA Rules, the rules of any other SRO or securities laws. This podcast is provided as is. FINRA and its affiliates are not responsible for any human or mechanical errors or omissions. Parties may not reproduce these podcasts in any form without the express written consent of FINRA.